
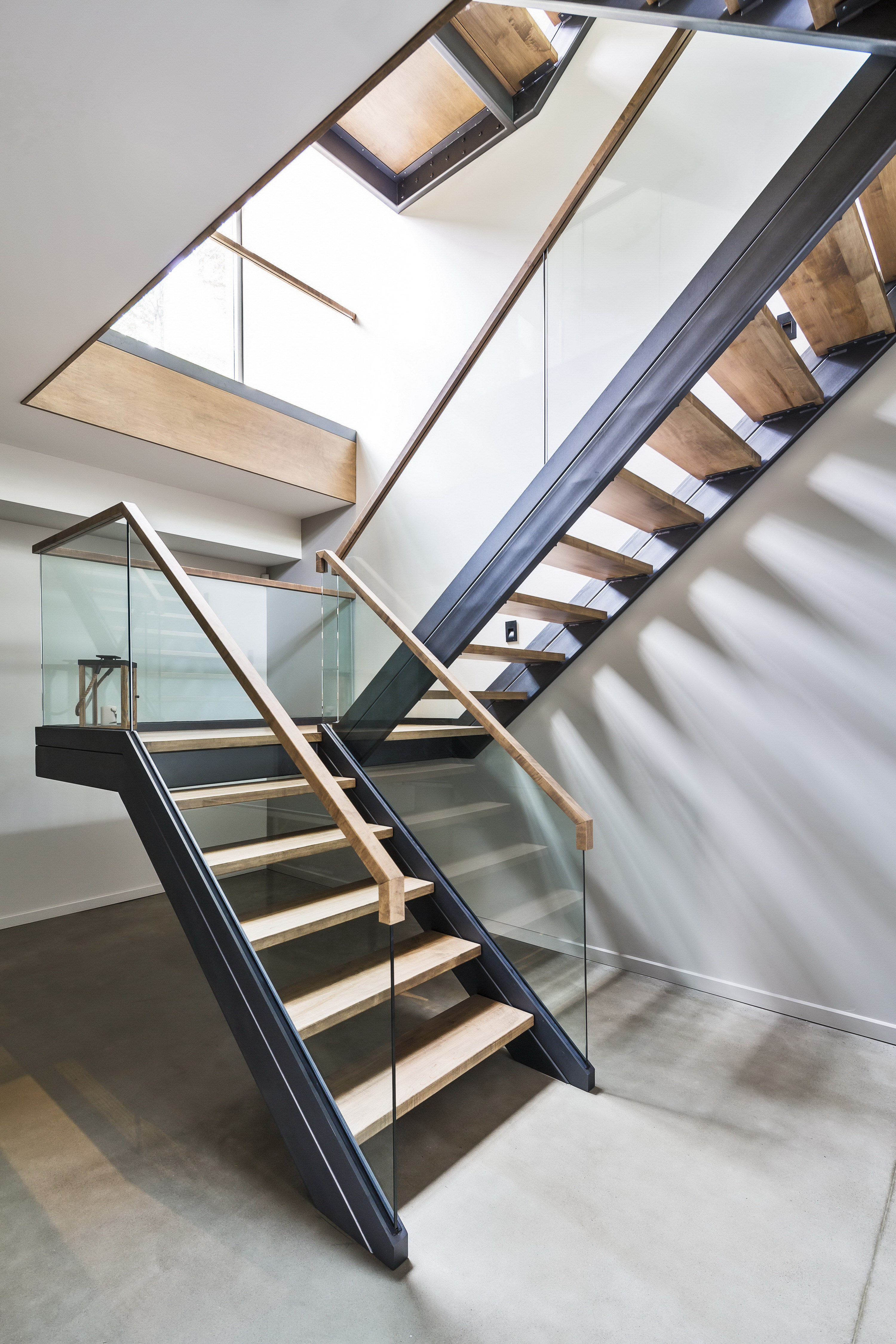
This also includes support for raw and unstructured data, like audio and video.Īccording to S&P Global Market Intelligence, the first documented use of the term “data lakehouse” was in 2017 when software company Jellyvision began using Snowflake to combine schemaless and structured data processing. Data lakes often require a data engineer to “wrangle” the data into a usable format.Ī data lakehouse, however, has the data management functionality of a warehouse, such as ACID transactions and optimized performance for SQL queries. While business analytics teams are typically able to access the data stored in a data lake, there are limitations. How do data lakehouses compare to data warehouses?ĭata warehouses are built for queryable analytics on structured data and certain types of semi-structured data. A lakehouse solves this problem by automating compliance processes and even anonymizing personal data if needed.
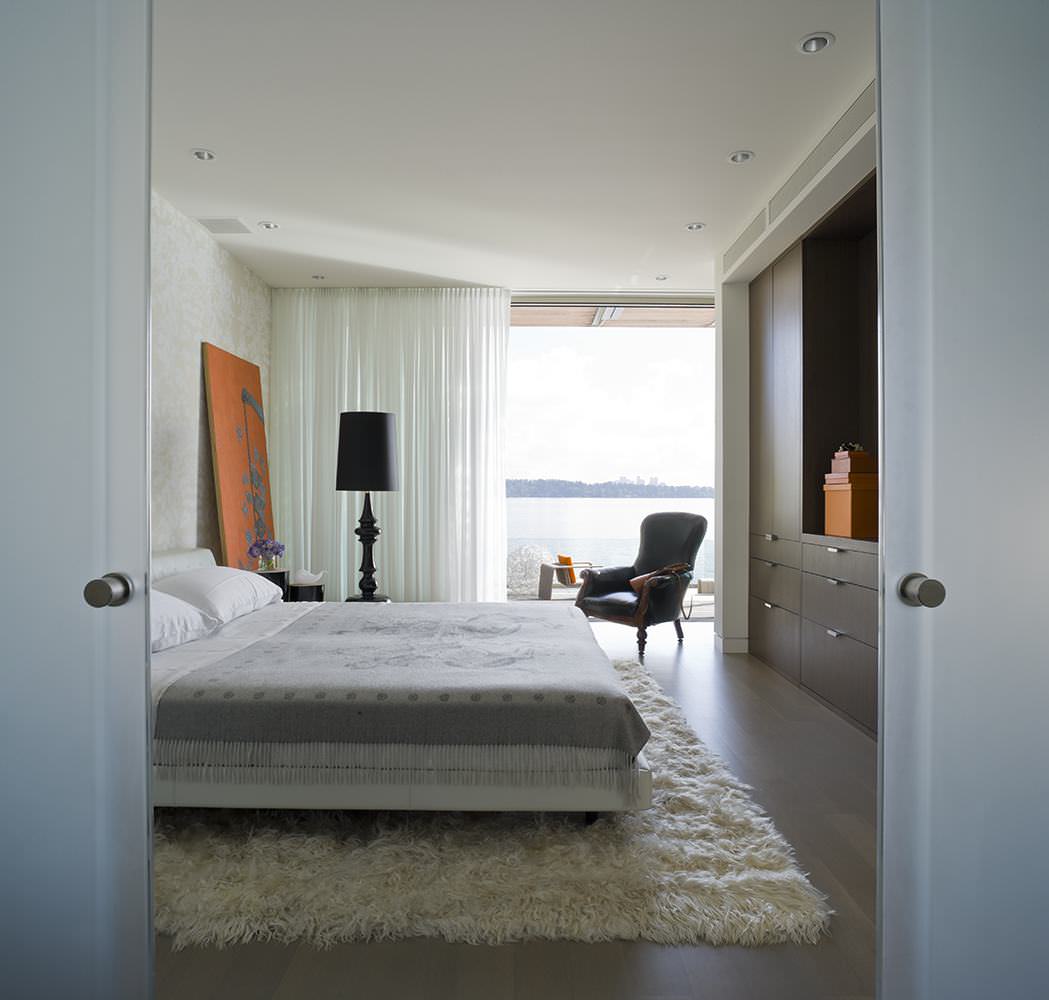
Though the unstructured data needed for AI and ML can be stored in a data lake, it creates data security and governance issues. In fact, lakehouses enable businesses to use BI tools, such as Tableau and Power BI, directly on the source data, resulting in the ability to have both batch and real-time analytics on the same platform.ĭata lakehouses also give businesses the ability to adopt AI and machine learning (ML) or take their existing technology to the next level, while still meeting compliance requirements. Decreased cost: By streamlining ETL processes and moving to a single-tier architecture, businesses often save money after adopting the data lakehouse approach.įeaturing increased agility and up-to-date data, it’s clear that data lakehouses are a great fit for organizations looking to fuel a wide variety of workloads that require advanced analytics capabilities.Fresher data: The issue of data staleness is addressed with a data lakehouse because data is available for analysis in a few hours rather than a few days.Reduced data redundancy: The data lakehouse serves as a single repository for all data, eliminating redundancies and supporting more efficient data movement.Improved reliability: Businesses don’t have to worry about engineering ETL transfers between fragile systems that may be disrupted due to quality issues.This simplified data infrastructure solves several challenges that are inherent to the two-tier architecture mentioned above: Why might a business use a data lakehouse?Ĭombining data lakes and data warehouses into data lakehouses allows data teams to operate swiftly because they no longer need to access multiple systems to use the data. Consumption layer: The business tools and applications that leverage the data stored within the data lake for analytics, BI, and AI purposes.
#LAKEHOUSE ARCHITECTURE HOW TO#
API layer: Metadata APIs allow users to understand what data is required for a particular use case and how to retrieve it.The metadata layer is the defining element of the data lakehouse. This enables data indexing, quality enforcement, and ACID transactions, among other features. Metadata layer: A unified catalog that provides metadata about all objects in the data lake.Storage layer: Various types of data (structured, semi-structured, and unstructured) are kept in a cost-effective object store, such as Amazon S3.Ingestion layer: Data is pulled from different sources and delivered to the storage layer.To address the data storage aspect, a relatively new open source standard called Delta Lake brings the essential functionality of a data warehouse, such as structured tables, into a data lake.ĭata lakehouse architecture is made up of 5 layers: Pioneered by Databricks, the data lake house is different from other data cloud solutions because the data lake is at the center of everything, not the data warehouse. Benefitting from the cost-effective storage of the data lake, the organization will eventually ETL certain portions of the data into a data warehouse for analytics purposes.Ī data lakehouse, however, allows businesses to use the data management features of a warehouse within an open format data lake. As a result, these organizations typically leverage a two-tier architecture in which data is extracted, transformed, and loaded (ETL) from an operational database into a data lake. When businesses use both data warehouses and data lakes - without lakehouses - they must use different processes to capture data from operational systems and move this information into the desired storage tier.
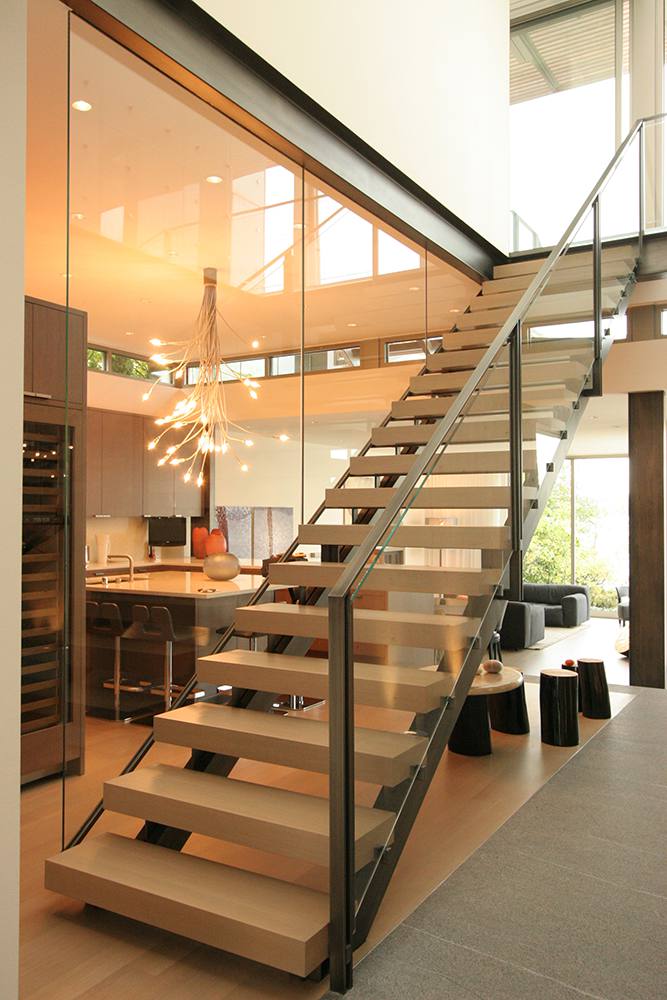
What are the components of data lakehouse architecture? A data lakehouse is an emerging system design that combines the data structures and management features from a data warehouse with the low-cost storage of a data lake.
